Christopher M. Pierce
Accelerator Physicist, xLight Inc.
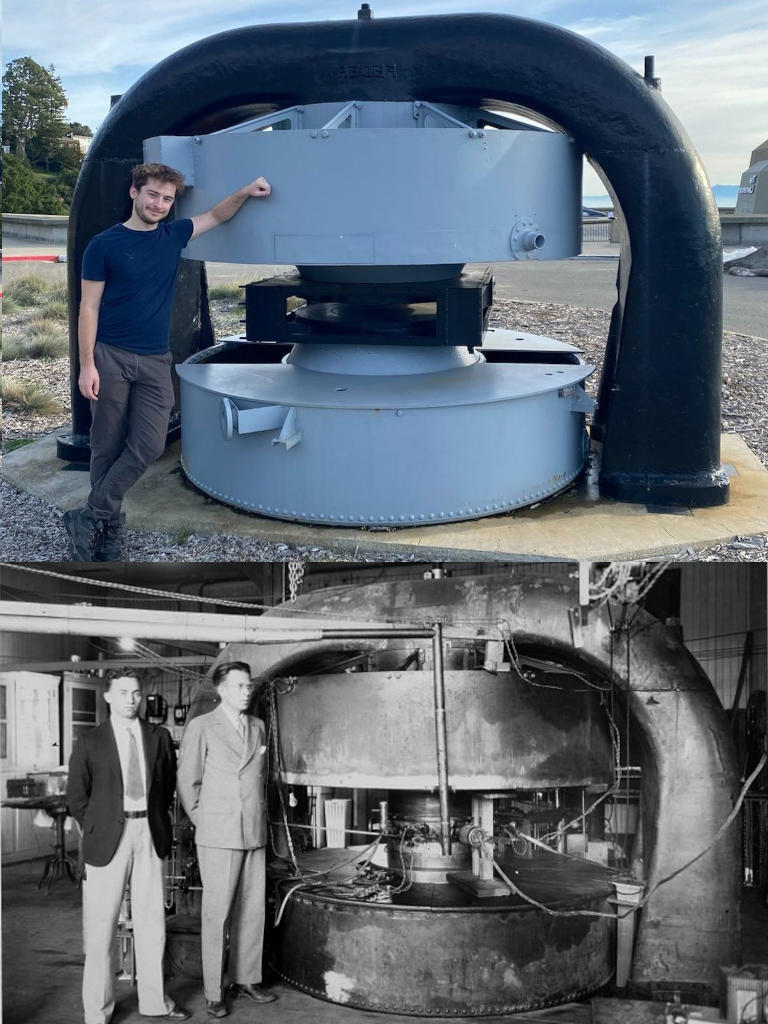
Me, in front of the magnet from EO Lawrence's 27-inch cyclotron
(photo credit: WH McNeil)
Particle accelerators are an ideal playground to explore the potential of scientific machine learning. These systems generate large, complex, and physically-constrained data sets, which can be challenging to analyze using traditional methods. Machine learning techniques, however, have shown promise in uncovering complex nonlinear patterns and correlations in data and we may be able to use them to improve the way particle accelerators are designed and operated. In my work, I am integrating machine learning techniques with the physical laws that govern charged particle beams to improve the modeling, instrumentation, control, and optimization of particle accelerators.
As an example of the power of machine learning, the following figure represents the output of a neural network which has been trained to predict the shape of a particle beam in phase space as it traverses a toy particle accelerator. Nonlinear space charge forces degrade the beam’s “emittance” (in plain language, quality) over time. Space charge forces are usually expensive to model in physics-based simulation tools. However, after the neural network has been trained on sparse calculated results, it is able to query the properties of the beam as system parameters are varied in an efficient and differentiable manner.
In addition to my work on scientific machine learning, my interests include photocathode physics for particle accelerator applications. Photocathodes are used to generate high-brightness electron beams which are necessary for some applications of particle accelerators, such as free electron lasers and ultrafast electron diffraction. The brightness of electron beams produced by the photocathode is highly dependent on the choice of photocathode material and in particular a material-dependent property called the mean transverse energy. Measuring the mean tranverse energy of photocathodes and devloping models that can predict this metric based on other material properties and understand how real-world problems can affect models are important goals in accelerator physics.
My work in the area of photocathode physics is related to exploiting the electronic band structure of semiconductors to limit the mean transverse energy of the photocathode and explore nonlinear photoemission processes via nanopatterned surfaces as a mechanism to shrink the photocathode’s source size (see image below). My past work has also shown how nonlinear processes can be enhanced using nanoscale patterning of metal surfaces to improve the efficiency of generating short pulses of electrons. Additionally, I created and maintain an open repository of published data related to photocathode materials. The Photocathode Database exists to connect researchers with published measurements on a variety of photocathode materials. Although it is currently small, I hope it will continue to grow in usefulness as I continue to digitize and add data to it. I am always looking for help to maintain this resources and interested parties should contact me for information on how to get started.
news
Jun 01, 2025 | For those with needs to estimate covariance/centroid from images of charged particle and laser beams, check out my library Beamfit available now through pip and conda ({conda, pip} install beamfit ). |
---|---|
Apr 24, 2025 | Check out my latest article out now in Swarm and Evolutionary Computation. A “comparison relationship surrogate” model is shown to improve the efficiency of a multi-objective genetic optimizer. The new algorithm (CRSEA) was measured to outperform many contemporary surrogate assisted optimizers on a wide variety of biobjective, medium scale problems. Find it on the journal’s website or see its preprint available on Arxiv. |
Dec 09, 2024 | Excited to announce the release of ParetoBench, a Python library for multi-objective optimization algorithm benchmarking. The package includes common test problems, tools for statistical analysis of convergence results, and objects for manipulating and saving results from benchmarking experiments. Go ahead and check it out on Github! |
Oct 28, 2024 | Pleased to have contributed to “Harnessing Plasmonic Interference for Nanoscale Ultrafast Electron Sources” out now in Physical Review Letters. The work demonstrates a novel approach to creating nanoscale electron sources using plasmonic focusing, achieving sub-40 pm-rad normalized transverse emittance. |
Aug 29, 2024 | Excited to announce the release of my new VSCode extension for the Bmad accelerator simulation environment! This extension enhances the development experience with features like syntax highlighting. Check it out on GitHub! |
Mar 03, 2024 | On my way to Korea for the ICFA ML Applications for Particle Accelerators Mini Workshop. Come say hi to me there! |
Dec 29, 2023 | I have completed all of the problems in Advent of Code this Year! Check out my solutions on Github. |
Nov 21, 2023 | Our conference proceeding “Kernel Density Estimators for Axisymmetric Particle Beams” has been published! Read more about it at the publication’s website. |
Sep 01, 2023 | I am happy to share that I am starting a new position as an accelerator physicist at a stealth startup. Looking forward to when I can share more about the exciting work we are doing! |
Jun 19, 2023 | Excited to present my work on physics-informed surrogate modeling for accelerators at Physics and Applications of High Brightness Beams! |
Mar 10, 2023 | Check out my latest publication in Physical Review Applied: Experimental Characterization of Photoemission from Plasmonic Nanogroove Arrays |
Jan 25, 2023 | I just launched a website! The Photocathode Database will connect researchers with published measurements on photocathode properties. |
Nov 02, 2022 | Say hi to me at the ICFA Beam Dynamics Mini-Workshop on Machine Learning Applications for Particle Accelerators! I am there presenting some of my work on how surrogate models can be installed into genetic algorithms and greatly improve the efficiency of multi-objective optimizations of accelerators. |
Nov 01, 2022 | I have joined the University of Chicago as a postdoctoral scholar and will be working with Young-Kee Kim on scientific machine learning. |
Oct 11, 2022 | The final paper of my Ph.D. is up on arXiv! This is some interesting work where we characterize, for the first time, the brightness of nanogroove enhanced photoemitters with applications for high average current photoinjectors. Check it out here: https://arxiv.org/abs/2210.05056. |
Oct 07, 2022 | My defense was today and I successfully presented on my thesis titled “Towards High Brightness from Photocathodes.” |